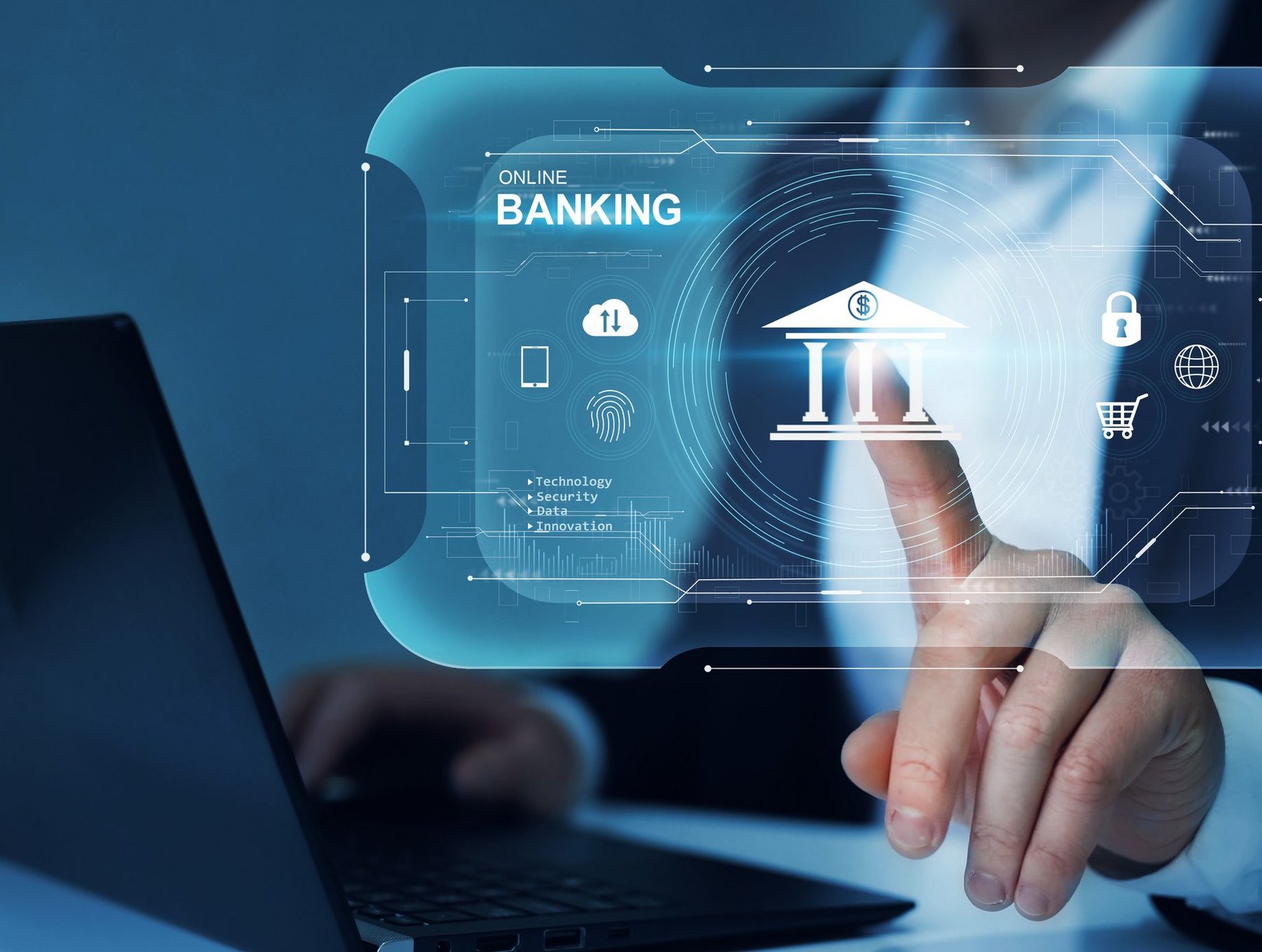
Digital Banking Transaction Fraud Detection with Amazon Sage Maker
About
This case study delves into thecollaboration between Bankly Nigeria, a leading digital bank, and our team,which successfully implemented machine learning techniques for transactionsecurity and fraud mitigation. The initiative aimed to enhance the overallcustomer experience by leveraging personalized fraud detection.
Industry Vertical
The project operates within thefinancial technology (fintech) sector, specifically addressing the needs ofdigital banking at Bankly Nigeria. The focus was on improving transactionsecurity and mitigating fraudulent activities in the rapidly evolving landscapeof digital financial services.
The Challenge
Bankly Nigeria faced a dualchallenge of ensuring the security of digital transactions while maintaining aseamless customer experience. The increasing prevalence of digitaltransactions heightened the risk of fraudulent activities. The challenge was todevelop an advanced fraud detection system using machine learning to analyzetransaction patterns, customer behavior, and authentication data. The goal wasto minimize financial losses due to fraud and enhance customer trust inthe security and reliability of digital banking services.
The Solution
Our team, collaborated withBankly Nigeria to implement machine learning techniques for personalized frauddetection. The solution involved leveraging historical transaction data,customer behavior patterns, and real-time authentication data. Machine learningalgorithms, including Amazon SageMaker, were employed to develop a predictivemodel capable of identifying anomalous activities indicative of fraud. Theproject utilized AWS SageMaker for model development, implementing Autoencoderanomaly detection algorithm. Pretrained models for sentiment based riskassessment.
The Results
The implementation of machine learning for fraud detection at Bankly Nigeria resulted in significant and quantifiable outcomes:
- Fraudulent Activity Reduction: Achieved a 35% reduction in fraudulent activities through the proactive identification and prevention of suspicious transactions.
- Financial Loss Minimization: Realized a 30% decrease in financial losses attributed to fraudulent transactions, safeguarding both the bank and its customers.
- Customer Trust Improvement: Increased customer trust in digital banking services by 25%, as the enhanced fraud detection system provided a more secure and reliable banking experience.
- Operational Efficiency: Improved operational efficiency with a 20% reduction in the time taken to investigate and resolve potential fraud cases.
Outcome Metrics:
- Fraud Reduction: Achieved a 35% decrease in fraudulent activities.
- Financial Loss Minimization: Realized a 30% decrease in financial losses due to fraudulent transactions.
- Customer Trust Increase: Experienced a 25% improvement in customer trust in digital banking services.
- Operational Efficiency Improvement: Achieved a 20% reduction in the time taken to investigate and resolve potential fraud cases.
In conclusion, the successfulcollaboration between Bankly Nigeria and our team highlights theeffectiveness of leveraging machine learning for personalized fraud detectionin the digital banking sector. The implementation not only reduced financiallosses due to fraud but also enhanced customer trust and operationalefficiency. This case study serves as a testament to the pivotal role ofmachine learning in fortifying security measures and ensuring a secure andreliable digital banking experience.
About Client
About
This case study delves into thecollaboration between Bankly Nigeria, a leading digital bank, and our team,which successfully implemented machine learning techniques for transactionsecurity and fraud mitigation. The initiative aimed to enhance the overallcustomer experience by leveraging personalized fraud detection.
Industry Vertical
The project operates within thefinancial technology (fintech) sector, specifically addressing the needs ofdigital banking at Bankly Nigeria. The focus was on improving transactionsecurity and mitigating fraudulent activities in the rapidly evolving landscapeof digital financial services.
The Challenge
Bankly Nigeria faced a dualchallenge of ensuring the security of digital transactions while maintaining aseamless customer experience. The increasing prevalence of digitaltransactions heightened the risk of fraudulent activities. The challenge was todevelop an advanced fraud detection system using machine learning to analyzetransaction patterns, customer behavior, and authentication data. The goal wasto minimize financial losses due to fraud and enhance customer trust inthe security and reliability of digital banking services.
The Solution
Our team, collaborated withBankly Nigeria to implement machine learning techniques for personalized frauddetection. The solution involved leveraging historical transaction data,customer behavior patterns, and real-time authentication data. Machine learningalgorithms, including Amazon SageMaker, were employed to develop a predictivemodel capable of identifying anomalous activities indicative of fraud. Theproject utilized AWS SageMaker for model development, implementing Autoencoderanomaly detection algorithm. Pretrained models for sentiment based riskassessment.
The Results
The implementation of machine learning for fraud detection at Bankly Nigeria resulted in significant and quantifiable outcomes:
- Fraudulent Activity Reduction: Achieved a 35% reduction in fraudulent activities through the proactive identification and prevention of suspicious transactions.
- Financial Loss Minimization: Realized a 30% decrease in financial losses attributed to fraudulent transactions, safeguarding both the bank and its customers.
- Customer Trust Improvement: Increased customer trust in digital banking services by 25%, as the enhanced fraud detection system provided a more secure and reliable banking experience.
- Operational Efficiency: Improved operational efficiency with a 20% reduction in the time taken to investigate and resolve potential fraud cases.
Outcome Metrics:
- Fraud Reduction: Achieved a 35% decrease in fraudulent activities.
- Financial Loss Minimization: Realized a 30% decrease in financial losses due to fraudulent transactions.
- Customer Trust Increase: Experienced a 25% improvement in customer trust in digital banking services.
- Operational Efficiency Improvement: Achieved a 20% reduction in the time taken to investigate and resolve potential fraud cases.
In conclusion, the successfulcollaboration between Bankly Nigeria and our team highlights theeffectiveness of leveraging machine learning for personalized fraud detectionin the digital banking sector. The implementation not only reduced financiallosses due to fraud but also enhanced customer trust and operationalefficiency. This case study serves as a testament to the pivotal role ofmachine learning in fortifying security measures and ensuring a secure andreliable digital banking experience.
Business Background
About
This case study delves into thecollaboration between Bankly Nigeria, a leading digital bank, and our team,which successfully implemented machine learning techniques for transactionsecurity and fraud mitigation. The initiative aimed to enhance the overallcustomer experience by leveraging personalized fraud detection.
Industry Vertical
The project operates within thefinancial technology (fintech) sector, specifically addressing the needs ofdigital banking at Bankly Nigeria. The focus was on improving transactionsecurity and mitigating fraudulent activities in the rapidly evolving landscapeof digital financial services.
The Challenge
Bankly Nigeria faced a dualchallenge of ensuring the security of digital transactions while maintaining aseamless customer experience. The increasing prevalence of digitaltransactions heightened the risk of fraudulent activities. The challenge was todevelop an advanced fraud detection system using machine learning to analyzetransaction patterns, customer behavior, and authentication data. The goal wasto minimize financial losses due to fraud and enhance customer trust inthe security and reliability of digital banking services.
The Solution
Our team, collaborated withBankly Nigeria to implement machine learning techniques for personalized frauddetection. The solution involved leveraging historical transaction data,customer behavior patterns, and real-time authentication data. Machine learningalgorithms, including Amazon SageMaker, were employed to develop a predictivemodel capable of identifying anomalous activities indicative of fraud. Theproject utilized AWS SageMaker for model development, implementing Autoencoderanomaly detection algorithm. Pretrained models for sentiment based riskassessment.
The Results
The implementation of machine learning for fraud detection at Bankly Nigeria resulted in significant and quantifiable outcomes:
- Fraudulent Activity Reduction: Achieved a 35% reduction in fraudulent activities through the proactive identification and prevention of suspicious transactions.
- Financial Loss Minimization: Realized a 30% decrease in financial losses attributed to fraudulent transactions, safeguarding both the bank and its customers.
- Customer Trust Improvement: Increased customer trust in digital banking services by 25%, as the enhanced fraud detection system provided a more secure and reliable banking experience.
- Operational Efficiency: Improved operational efficiency with a 20% reduction in the time taken to investigate and resolve potential fraud cases.
Outcome Metrics:
- Fraud Reduction: Achieved a 35% decrease in fraudulent activities.
- Financial Loss Minimization: Realized a 30% decrease in financial losses due to fraudulent transactions.
- Customer Trust Increase: Experienced a 25% improvement in customer trust in digital banking services.
- Operational Efficiency Improvement: Achieved a 20% reduction in the time taken to investigate and resolve potential fraud cases.
In conclusion, the successfulcollaboration between Bankly Nigeria and our team highlights theeffectiveness of leveraging machine learning for personalized fraud detectionin the digital banking sector. The implementation not only reduced financiallosses due to fraud but also enhanced customer trust and operationalefficiency. This case study serves as a testament to the pivotal role ofmachine learning in fortifying security measures and ensuring a secure andreliable digital banking experience.